Over the past decade in-store analytics tools have been a transformational technology for retailers and brands of all sizes. For example, category managers can analyze every single shopper touchpoint but I still hear a lot of confusion about how to get the best out of data management and analytics.
In this article I will describe some of in-store analytics’ best practices you should bear in mind – if you like, discussion topics you can bring to the table when a broader of investing in big data technologies arises in your retail organization. Not overly technical in nature since data analytics is a business asset, not a technical resource. In fact let’s start there:
1. Focus on your business needs, not the technology
Technology, especially in the field of instore analytics, is advancing at a rapid pace. We can get carried away by the technology itself, assuming that if a new capability exists, there must be an advantage to using it.
For example, many of our customers tell us and other consultants that they want to do real-time analytics on data. But if we dig into what this means, we often find two problems that are not technical at all. First, data is generated and collected at a much finer level of detail than many retailers work with now. And second, even if data systems can deliver actionable analytics as data is collected or changes, the retailer is not equipped to make relevant decisions at that speed. One result is that retailers find their actions lagging behind the data analysis, which means they have, to a certain extent, spent unnecessary costs. Such a mismatch between the flow of data and the cadence of retail business decisions can also leave users feeling stressed and overloaded with information that gets in the way of doing a job well.
“When we deal with requests for real-time analytics, we think it’s worth asking whether “right-time analytics” would better suit the rhythm of the retailer”.
2. Collecting lots of data is a good thing, not a problem
Many retailers managers complain of feeling overwhelmed by data and see data as part of the problem. For sure, you shouldn’t swamp even experienced analytics professionals with more data that they can comfortably take in and make sense of.
Nevertheless, not all data has to be analyzed by humans. Machine learning algorithms and retail enterprise AI tools can take advantage of big data volumes that data science teams couldn’t handle on their own. Down the road there will be patterns in the historical data dat can be used to detect potential business problems or opportunities. We can help you deliver alerts and notifications to help improve business decisions.
“We don’t let data overwhelm you. We help you focus on effectively delivering the most appropriate analytics for decision-making now, while also storing, governing and managing data for use cases and analytics scenarios you may not even know about yet”.
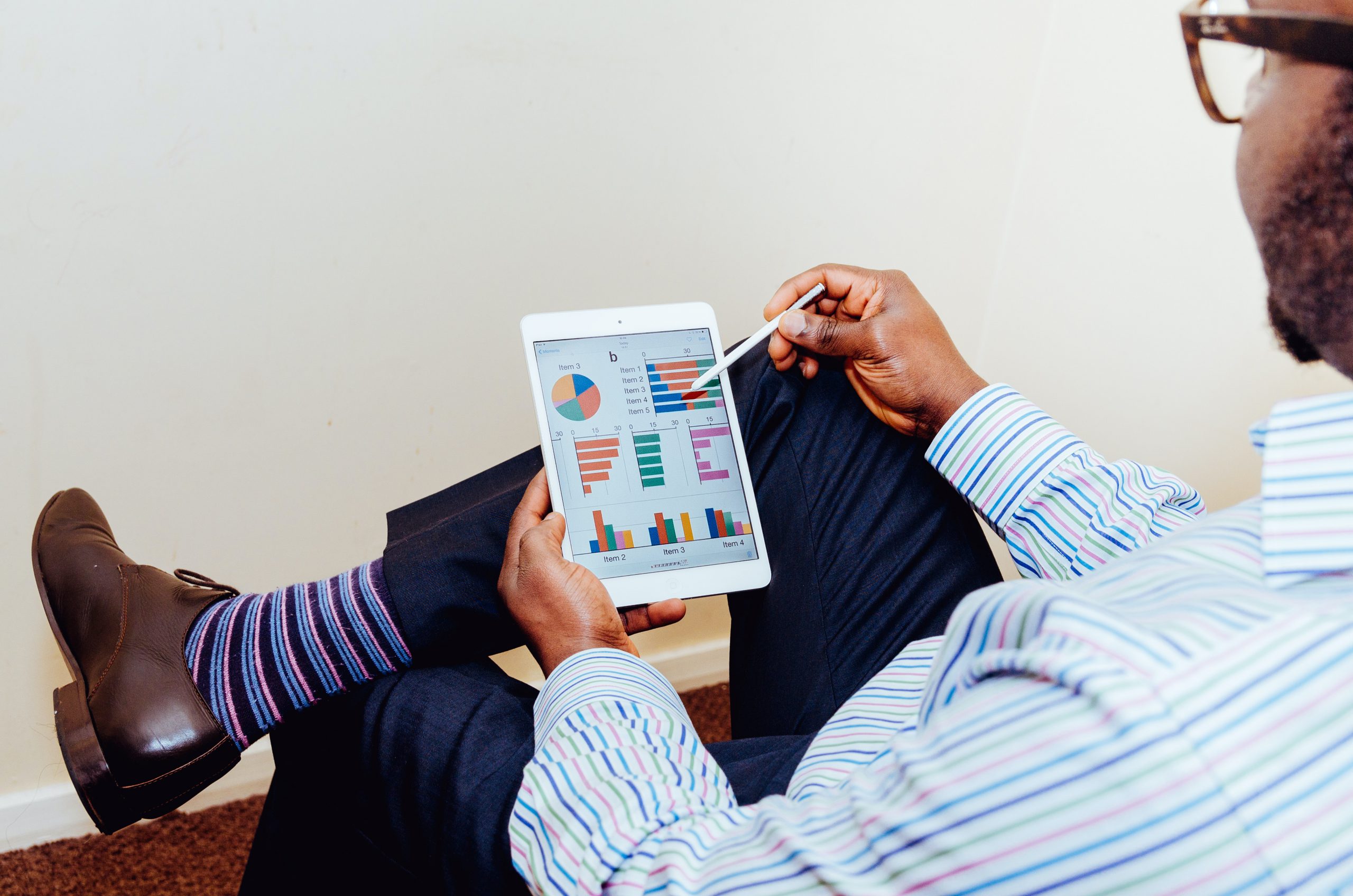
3. Use data visualization to enable data discovery and analysis
When working with information at scale, our visual capacity is unmatched for making sense of it all. Even colleagues who don’t have the coding skills to write a clustering algorithm (me neither) can easily pick out a clutch of close data points in a chart generated by an algorithm. Spotting a few values that just don’t fit into the visual pattern is easy.
“With appropriate data visualizations we make you all natural data analysts. We’ll help you to create well-designed visual representations of the data and analytic results”.
4. Iterate on structuring data to match specific applications
Future proofing data lakes and data platforms is extremely important. If you leave the data in its native format, you don’t need to structure the data first. Over time, when you discover new uses for sets of data as your analytics team develops, business needs and technology advances data can be filtered, transformed and organized as needed or each new analytics application.
“We’ll help you run the marathon, not a sprint. Such an iterative approach should be an essential component of your long-term strategic thinking of in-store analytics”.
5. Consider the cloud for deployments of big data systems
You may worry about the costs of keeping so much data around. Rather than being an expensive barrier to your data strategy, cloud services can really help. For one thing, cloud platform vendors price data storage as a commodity, typically making it far cheaper than buying your own on-premises storage devices. In addition, they manage data security, availability, backup and restore, replication and archiving on your behalf.
“We’ll help you save money and execute the best possible data strategy”.
6. In today’s regulatory environment, strong data governance is no longer optional
In today’s regulatory environment, strong data governance is no longer optional: it must be a primary consideration in your data strategy.
“Working with us ensures you not to break the law but well-governed data is worth so much more. Well governed-data is also a better resource for data analytics and easier to work with, too”.
In today’s regulatory environment, strong data governance is no longer optional: it must be a primary consideration in your data strategy. “Working with us ensures you not to break the law but well-governed data is worth so much more. Well governed-data is also a better resource for data analytics and easier to work with, too”.
Want to put these best practices into action?
We have seen again and again, technologies are not enough on their own. Data is a business asset, without business-focussed analytics, it may be a wasted one. We’ll gladly share our experience and help you move forward.